For most companies, the adoption of the Internet of Things means opening the flood gates to a wave of sensor data that needs to be collected, processed, managed and acted upon. This is a daunting task, and done incorrectly it can lead to failed IoT implementations. We know that business intelligence (BI) tools help display information, but it is the underlying data analytics, founded on data science, that makes IoT data valuable and able to be acted upon to meet your business goals.
Let’s start with understanding the difference between business intelligence and data analytics, and then we can get into how this feeds into actionable data:
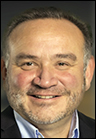
Business Intelligence
This refers to the Ts: tools and talent. BI tools convert business data into meaningful visuals, reports and dashboards that provide context for analysts and other subject-matter experts to make business decisions. BI can range from very general contextual awareness to very specific use cases; but ultimately, BI is focused on situational awareness.
BI can be a business performance enabler, but we need to recognize that it is not focused on solving specific business questions. It’s a relatively passive solution, limited to descriptive statistics, pivot table type queries and standard data visualizations.
Data Analytics is a much more sophisticated evolution of informing business decisions through applied math, data wrangling and subject-matter expertise. At the most fundamental level, data analytics is the application of data science toward solving business problems. Data analytics certainly provide an input into BI solutions. For example, one could build a data analytics solution that enriches business data (for example, through business rules and heuristics that leverage the subject-matter experts in my business) and generates a higher-quality data to feed into a BI dashboard.
Perhaps we are generating risk scores for specific components of a process, and feeding those risk scores into a BI tool so that analysts can check on the daily status of the system. Under the hood, this solution would include both a data analytics solution (generation of the risk scores) and a BI solution (descriptive dashboard of risk scores, perhaps depicting changes in last 24 hours).
The bottom line—BI tools are only as good as the data that is being presented, and this is where data science comes to the rescue.
Data Science
Data science has a foundation in applied mathematics. This does not mean that data science needs to be complex; many of the simplest approaches are often the most effective. But it does mean that there is real math being done on the data, and that the outcome of this approach ties the data output to your business needs.
This gets tricky when you want to ensure that the mathematical equations are representative of the actual business requirements, such as improving overall system efficiency to increase production rates, for example. We start by asking business questions and doing some initial research on the most appropriate approaches and methods.
The end result is a data analytics output that can reveal keys to improved business performance and actually make the output actionable. The challenge is in making sense of collected data and unlocking its value, and that is where both predictive and prescriptive analytics come in.
Most people recognize predictive analytics. Through machine learning, it is possible to model how a piece of equipment operates and identify the factors that influence its behavior. Once you understand the leading indicators to a failure event, you can then monitor for those conditions and take proactive action (i.e., the prediction). This is a powerful tool when applied to mission-critical equipment where unexpected downtime has a serious impact to the business.
Less attention has been given to prescriptive analytics. Leveraging the same data models that are used to understand equipment behavior, you can establish a desired baseline of performance and then compare that to real-time operations in the field. Not only does this enable you to identify where equipment output does not conform to a baseline, but this technique can provide a prescriptive remediation plan for bringing that equipment into compliance. With the right systems in place, you can take real-time actions to the equipment through automation and help reduce or eliminate unplanned downtime.
When thinking through your IoT strategy, using data science and analytics to make sense of the massive amount of data being generated will help you achieve your business objectives. It will maximize uptime and performance of critical corporate assets, help reduce operational expenses and increase business efficiency. This is what we call “The Business of IoT.”
Dave Wagstaff is the chief technology officer of Bsquare, an IoT solutions provider. In this role, Wagstaff drives a comprehensive and integrated strategy for all Bsquare products, including DataV, the company’s flagship product. He has held a number of senior and strategic technology positions, including chief architect of advanced solutions for Lantroni; director of engineering at Lantronix; director of software development at Open Text; and software development manager at both Gauss Interprise and Diebold.