Cognosos has released a real-time location system (RTLS) for hospitals that employs artificial intelligence (AI) and machine learning (ML) to identify the specific room in which a Bluetooth Low Energy (BLE) tag is located. This, according to Adrian Jennings, the company’s chief product officer, enables the low-cost and non-disruptive installation of an asset-management system without requiring additional sensors in each patient room.
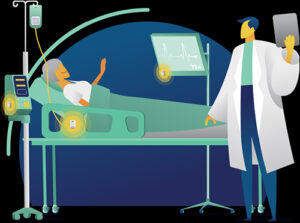
Cognosos’s RTLS technology enables the non-disruptive installation of an asset-management system at healthcare facilities.
The Atlanta technology startup provides AI-based location data. Its LocationAI platform serves as a machine learning location engine for asset tracking at hospitals and other healthcare facilities. The solution includes BLE devices that transmit data to a self-healing network in order to understand each device’s location at the room level, and it monitors and responds to environmental changes, such as new furnishings, or infrastructure that could affect transmission.
Additionally, Cognosos is releasing a feature that employs LocationAI data to help hospitals ensure that assets move appropriately through clean rooms, and that they are staged and ready for use, according to each hospital’s specific needs. The ProPAR solution (an acronym for Proactive Periodic Automated Replenishment), released on Apr. 11, includes a suite of features and dashboards designed to support central supply departments and biomedical engineering teams by providing visibility and alerts related to asset counts and PAR level thresholds.
Intelligence-based Localization
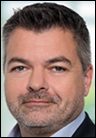
Adrian Jennings
LocationAI takes a new approach to RTLS for the healthcare market, as it relies more on software and intelligence, rather than an infrastructure of receivers or beacons, to know where each tagged item is located. Its ML algorithms are what identify the room in which objects can be found. This is what Jennings—who has been in the RTLS business for several decades—calls a completely fresh approach to RTLS technology. He joined Cognosos in its early development stage, approximately a year ago.
Speaking of the company and its development process, Jennings says, “When you’re a newcomer to the industry, you’re not weighted down with the ‘This is how it’s done’ approach.” The software platform’s AI and ML functionality are designed to calculate a tag’s location in the same way that an image-recognition algorithm can be trained to recognize specific images. LocationAI learns and infers whether an asset is in one room as opposed to another.
Challenges of RTLS
Standard RTLS solutions, Jennings says, have been aimed at calculating the XY and sometimes Z coordinates, via a network of transmitters around a facility, and on creating software-defined boxes to delineate rooms within that network. A dot representing a tag appears in the software, inside a specific room, based on data collected from the hardware. “The whole premise of RTLS has been ‘Let’s figure out the precise location of a thing via triangulation and tri-laterization, and then we can infer useful information from that,'” Jennings states.
However, without very precise data, a tag near a wall in one room could be perceived to be in the next room, or even on the next floor. Using infrared or ultrasound is one option to gain more granular location data, with a sensor installed inside each room. If a tag detects a sensor signal, the system knows the room in which it is located. But that requires a large amount of added infrastructure, Jennings says, and installing sensors in every room could be disruptive to patients and healthcare providers. “That’s a big problem,” he adds. “You can’t just disrupt the workflow in a busy hospital to install infrastructure.”
Cognosos says it has approached the problem from a different position. The company had previously developed outdoor tracking technology that is used in the logistics sector for vehicle and trailer tracking with very lightweight infrastructure. That solution, Jennings says, is easy to deploy and relatively low in cost. “The question,” he recalls, “was, could we do that in hospitals?”
With the system, a beacon device mounted in the hospital transmits its ID. The Cognosos tags, built from standard off-the-shelf BLE chipsets, are applied to each asset, such as a hospital bed, an IV pump or a wheelchair, and they receive the beacon’s transmission. The tag then forwards the data to a gateway via a proprietary active RFID frequency. The gateway sends the data to the server, on which the system’s ML and AI calculate the details and, based on previous reads, surmise that BLE tag’s location.
To provide the base data on which the machine learning operates, users would first need to move the device through rooms so that information could be captured and associated with the rooms in which the tags are beaconing. The software can thus identify the characteristics of a transmission from each room. This could be performed by nurses or housekeeping personnel as they go into and out of patient rooms. “There’s all kinds of opportunities to actually calibrate the system,” Jennings says.
Preventing Information Decay
According to the company, a fairly sparse number of low-cost Bluetooth beacons installed down a hallway would be sufficient to gain, with high confidence, data regarding the room in which a transmitting tag is located. How the beacons are installed is unimportant, Jennings says. “In fact,” he states, “the more randomly they’re placed,” the better the system can identify characteristics of transmissions from each room.
Because the ML system constantly updates itself, Cognosos notes, it prevents the decay of location data that can occur in standard RTLS solutions. If the environment changes, such as a new piece of furniture being added—which would change transmissions—the system can identify and learn the details of the updated environment. “The beautiful thing with machine learning is it never stops learning,” Jennings says, “so every day, many times a day, we get updates and we can use those to see something has changed.”
Beacons are placed about every 30 to 40 linear feet, or one every 1,000 to 2,000 square feet, to provide full area coverage. Cognosos provides the BLE tags and beacons, along with the gateways. Typically, the company indicates, one or two gateways would be sufficient to cover a hospital floor.
Transmissions from beacons back to a gateway are accomplished via a proprietary RF frequency, Jennings says, because the datalink needs to be non-disruptive with other wireless networks at a typical hospital, as well as low in cost. Once the information is transmitted to the gateway, the latter forwards it to a cloud-based server, where the AI and ML functionality take place. “We do all of the hard work in the cloud,” he says.
The system includes the new ProPar feature to help users understand the conditions in supply rooms, based on locations of equipment without having to sign into a dashboard to search for those items. The software tracks data regarding equipment location, as well as when it exceeds a pre-determined dwell time at any given location, or a number of similar assets at one location. The software can then alert asset-management personnel with a list of items that need to be collected from one site and moved to another.
“It’s making the clean room intelligent,” Jennings says. “The clean room actually reaches out and asks to be replenished when it knows that its stocks are falling.” In the long run, he predicts the system will enable hospitals to reduce the amount of redundant equipment they purchase and keep on-hand for redundancy.
Key Takeaways:
- AI and machine learning offer an alternative to standard RTLS technology that could reduce infrastructure and deployment costs.
- BLE beacons with proprietary RF functionality provide the data needed to help AI and machine learning calculate a location.
Exhibitors at RFID Journal LIVE! 2022 will offer solutions utilizing artificial intelligence, machine learning and Bluetooth Low Energy. To learn more, visit the event’s website.