Researchers at the MIT Media Lab are employing radio frequency identification technology along with computer vision to enable robots to explore their environment in order to locate and move a targeted item that may not be visible. The system, which has been in development, simulation and testing for several years, employs machine learning to better accomplish such complex tasks, and the team is seeking to commercialize the research.
In that effort, the researchers have been interviewing potential customers and planning a possible company spinoff. This year, the team has participated in the I-Corps program, led by the National Science Foundation to identify potential sponsors and plan the first product. “The technology has matured enough to take it out of the lab into the real-world environment,” says Fadel Adib, an MIT associate professor and the Media Lab’s principal investigator.
The RFID portion of the robotic system employs what researchers call RF perception, consisting of off-the-shelf passive UHF RFID tags, as well as an RFID reader and specialized antennas installed in the robot’s environment. Robots employ RFID to identify items and their specific locations when they are not visible, and the software analyzing that data can direct the robots via computer vision to focus on the items before them, determine what needs to be moved or navigated around, and act accordingly. The technology, the researchers say, could be leveraged by manufacturers, retailers or warehouses to sort, pick or place goods.
The robot is designed for two primary solutions, according to Adib. One is monitoring goods moving through warehouses that need to be picked and packed according to customer orders, which traditionally requires workers to move through aisles, opening boxes and finding specific items, then placing them in containers for shipping. With RFID, the robots could identify what is in a given box or on a particular shelf, then pick up that item and confirm where it was placed. The system is designed to prevent errors, which means companies could minimize the rate of goods being returned due to the wrong item having been shipped.
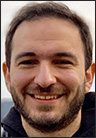
Fadel Adib
The other use case involves complex, crowded environments in fixed areas, such as a dedicated space where returned items are sorted and processed. The robot is designed to sort through a pile of products and identify them. It could move unneeded or lower-priority items out of the way and pick up the tagged item it seeks, then place it elsewhere, such as in a box for shipment.
Although many companies use robotics for the identification and movement of goods, Adib says, “What we’re focusing on is the last mile, the last meter, which is highly complex—places where you need to identify a specific item and grasp it.” Traditionally, robots have had trouble locating and gasping objects in crowded environments, says Tara Boroushaki, an MIT Media Lab research assistant and student lead of the RF-Grasp project. While computer vision can help a robot understand what is directly in front of it, if the goods it seeks are in a box or hidden by another object on a shelf, the robot becomes less reliable.
MIT Media Lab has been working with RFID technology, including the RFID and computer vision solutions, for four years (see MIT Media Labs Creates Highly Precise UHF RFID for Robotics and RFID Detects Food Safety with Innovation from MIT Media Lab Research). The lab’s TurboTrack system is designed to pinpoint a UHF RFID tag within less than a centimeter.
To accomplish highly granular localization, the system employs at least three RFID antennas, which transmit short-duration pulses at 800 to 900 MHz to a UHF reader that sends standard 902 to 928 MHz transmissions to interrogate tags. MIT Media Lab’s software then employs artificial intelligence to identify the specific location of each tag based on its responses to the interrogation and antenna pulses.
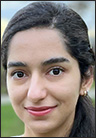
Tara Boroushaki
That earlier work on TurboTrack led to the latest project to leverage machine learning for computer vision and RFID, in order to help robots locate things the same way people do. The system tested by the lab consists of a robotic arm attached to a gasping hand with a camera at the wrist. Throughout the past year, Boroushaki says, the lab has been simulating machine learning to enable better management of data and thus ensure the robot can analyze both RFID and vision technologies in a fused manner.
In a typical deployment, the robot can use RFID to identify a targeted object’s location, then capture RGB-D (color and depth) images to create a camera-based 3D model of the environment. The software fuses the RFID location to that model, and the robotic arm moves within grasping range. It identifies the RFID-tagged item it is grasping and moves it to the appropriate location, then releases it.
With RFID, the robot can understand if it has picked up an item that does not have a tag attached (since the target tag will not be perceived as having moved), as well as if it has grasped the wrong item (since the incorrect RFID tag will move). The robot can set aside any item that it determines does not have the targeted tag ID.
Numerous companies are currently seeking solutions to locate goods robotically as a means of replacing the need for humans to select and move items. The robotic version, the researchers explain, will make operations more efficient and safer. The question, Boroushaki says, is how to enable a robot to find something it cannot see. She has been leading that effort since fall 2019 and completed the project, including lab testing, in October of last year.
MIT Media Lab first tested simulated environments during the COVID 19 pandemic, throughout four months of quarantine. “We developed a system that tries to avoid crashing into obstacles,” Boroushaki says, “and moves toward items in simulation.” When the researchers returned to the lab, they tested the solution on their robot and found that the machine-learning tools worked well. “Development was a combination of simulation, running on a real system,” she adds.
The project used Universal Robots‘ UR5 robotic arm, combined with an Intel camera. MIT designed and built its specialized RFID reader system using off-the-shelf RFID tags. The organization has begun discussing the technology with industry players, Adib reports, such as Toppan Printing and some primary users in the apparel industry, which could be the primary beneficiaries. The team expects to next launch pilots in the real world.
“Our approach to commercialization is like research,” Adib says. “It takes an agile approach to quickly experiment, iterate and adapt.” The team foresees the technology being used in manufacturing, retail and logistics, as well as eventually in consumers’ homes. The pandemic has accelerated the need for robotic management of the flow of goods through the supply chain, Adib adds, while also speeding up technology development to meet those demands.