Datamars has released a new textile-management solution with its RFID tag readers that employs machine learning (ML), which the firm says can train itself to disregard stray tag reads and thereby enable better read effectiveness. Several companies have been piloting the technology since late 2020, with full deployments taking place now. The system, known as RFAID, consists of Datamars’ Classifier algorithm that learns which reads are wanted and which should be disregarded.
Headquartered in Switzerland, Datamars provides RFID-based technology for animal identification, farm management and textiles, such as the tracking of flat linens as they undergo laundering services. The company’s textile solutions are designed for the hospitality, healthcare and industrial sectors, says Julien Buros, Datamars’ textile product and service director. Its UHF RFID readers are built to read tagged linens, whether they are soiled, in the laundering process or being returned to a customer cleaned and folded.
This environment is challenging for RF, Datamars explains, in part because tags applied to wet or dried goods on trolleys often must be read as they move quickly through a laundry facility. That means readers must identify moving textiles, even in bulk, without interrupting workflows and avoiding stray reads of tags that might be within range of a reader but are not part of a particular workflow being tracked. Datamars provides a variety of UHF RFID readers, as well as edge-based reader-performance software that is integrated with application-based software.
To address the risk of stray tag reads, Datamars and other RFID companies have traditionally relied on physical barriers that block RF field propagation before they can be captured by a stray tag. This can be labor-intensive and costly for each deployment, the company notes, since laundry facilities tend to differ in terms of size, infrastructure and layout. Therefore, building an RFID system to meet the needs of such an environment can be challenging.
Another common option, Buros says, is to set power and sensitivity thresholds to filter stray reads, but this comes with shortcomings as well. While users could manually set read distance thresholds to identify when tags fall outside of the read area, that function would need to be customized for each deployment, based on the space in which a given reader was installed and the type of UHF tags being read.
The development of machine learning is designed to enable software to recognize a “good tag” read from a stray tag. “By training the network with large amounts of data,” Buros states, “the system does not require mechanisms such as thresholds to be set.” ML is a form of artificial intelligence (AI) that enables RFID readers to learn and differentiate tag reads automatically without being specifically programmed to do so. Training datasets from Datamars help the ML software running directly on the reader to find common patterns and build a model to make its own decisions. That data can be shared with other readers in a network in order to provide further learning.
The system leverages the standard data that comes with each read event, including the returned signal strength indicator (RSSI), the signal phase, the number of tag reads accomplished within a specified amount of time and more. “If you look at all these parameters, you can start to recognize the good tag,” Buros explains. Over time, he says, based on the captured data, the system starts to identify the characteristics of stray reads, as opposed to the good tags.
By ensuring that the system recognizes and disregards stray reads, the machine learning function allows users to increase interrogation power, since the read distance threshold is not a concern, and even tags producing weak responses can be read. A key benefit of the ML functionality is that a deployment can be repeated across numerous sites, each with its own layout and use cases, and every reader can be trained to capture the appropriate information. As a result, each facility can make changes to its own operations as well, and the system can adapt without being reconfigured. This means less cost in RFID reader installation and manual configuration, Buros reports.
The more training is provided at each installation, the less is needed for additional training at another sites. “That’s what you call continuous learning,” Buros says, noting that the ML algorithm runs on the reader. Datamars employs Impinj Speedway and R700 readers. “We have enough computing power in both versions to run the algorithms,” he states. After several years of development and following in-house validation, Datamars provided the technology to customers for pilots late last year.
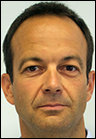
Julien Buros
At present, two companies are piloting the system—one for the past two months, another for five months. These businesses have achieved greater flexibility for their, Buros reports deployments, along with lower installation costs. “From a hardware point of view,” he says, “it’s a pretty light solution.” Since the system is more adaptable, a company could use the readers at multiple sites, each configured differently. “There are no two laundries that are alike.”
Datamars reports that it is among the first companies to leverage machine learning for RFID tag readers. “I think this could be a first step for laundry and textile identification with machine learning,” Buros says, while ML could be used similarly with other RFID solutions in different industries as well. “We do believe that to improve reading performance, you can do a lot with hardware, but there is also a lot of potential with software-based solutions.”
The ML solution to the stray-read problem in textiles follows the company’s release of a new family of RFID readers in 2019, known as the UHF Portal Plus and UHF Open Tunnel. The readers are part of the In Motion family, Buros says, intended to meet the challenges of laundry services environments (see UHF RFID Reader Systems Capture Laundry Tags in Motion).
The Portal Plus has been one of the company’s most successful textile-based products, Datamars reports, due to its ability to read tags without stopping the flow of products as they move through, into or out of a laundry facility on trolleys. The Open Tunnel reader is designed for fully automated facilities utilizing conveyors and feeding systems, such as automatic folding machines. The readers are being used to identify each textile item serviced and returned to a specific customer.
“It’s important for the laundry to be able to check that what is sent to its customer is correct,” Buros states. The data can also be shared with customers, he says, or to enable automated billing or loss prevention. “Now, with RFID, they are able to understand who is losing [products] and what is being lost.”