The Internet of Things (IoT) has already made a significant impact on the industrial and utility sectors, from smart meters to oil and gas monitors, and there is no arguing that it is changing the industry. With smarter technology, field-service managers are able to gain deeper insights that were not available in the past.
But the increased number of connected devices in the field also comes with a surge of data and an even bigger question: what do we do with all this data? As enterprises gather more data, they need to decide what type of information will be valuable for analysis and lead to decision-making impact—a key driving force for the next phase of growth for the Industrial Internet of Things (IIoT).
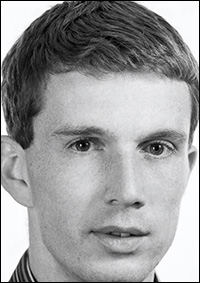
The first set of challenges organizations face involves where to store the data, whether to store all or just part of it, and how long to keep it for. Some people will say that cloud storage is cheap and, as a result, you should keep everything indefinitely, but overtime those costs will grow and eventually become significant enough to be questioned.
Techniques like downsampling can help reduce the amount of data while still allowing analysis. A sensor might capture a temperature reading every 10 seconds, but you might only want to keep it to a granularity of one reading per minute or even per hour. Those readings can be aggregated into one value, representing the average or middle value.
The next set of challenges are where and when to process the data. This is commonly done on a centralized system, but this approach usually has timeliness implications. Certain organizations have to turn to edge processing to avoid the delay it takes for data to flow back to a centralized system. Usually, the type of edge processing is kept quite simple, like raising alerts that need immediate action.
Manual data collection was typically carried out infrequently, such as every three months on a preventative maintenance schedule. This made it very difficult to see trends, but when data starts to be collected on a frequent interval every minute or few seconds, it opens up the opportunities to analyze trends and develop intelligence into analysis, real-time monitoring and alerting.
The final set of challenges to be discussed relate to real-time alerting based on readings collected. As we have seen in modern IT monitoring, IoT monitoring is likely to face the same problems around alert fatigue. This would be when a field technician receives too many false positive alerts and, as a result, begins ignoring them or becomes overworked.
Alert fatigue can happen when there is an over-reliance on raw readings being used in isolation. These raw metrics can be noisy and should not raise alerts unless correlating data matches certain conditions or unusual trends are identified. An alert will be raised if a machine’s temperature remains above a certain threshold for a few seconds, even though it may not be a problem that requires immediate attention, if there is a trend of that machine becoming hotter at a similar time each day and then having its temperature go back to normal. Knowing that trend may allow better categorization of that alert to be one lower in priority.
Data analysis and visibility for managers will drive the future of the IIoT. Visualization and data analysis can be categorized as either real-time or historical. Real-time analysis can be accomplished at the edge, often to raise alerts but also to aggregate multiple metrics or downsample. The downside with aggregation or downsampling at the edge is that you lose the ability in the central system to drill down. Historical analysis is more about examining trends and inferring what is likely to happen in the future.
In order to provide visualization for management, the data collected often needs to aggregate several times so that it can fit onto a single screen. They should always have the ability to drill down on those top-level metrics. You really want to keep the headline metrics to a minimum and make them as simple to understand as possible; otherwise, people will become overloaded and miss key indicators or changes.
Properly analyzed data from connected devices will have a huge impact on preventative maintenance, especially for industries that need to avoid breakdowns due to costly repairs involving oil wells and elevators, for example. Preventing all failures is not feasible in most cases, but analysis can also help to reduce the time required to restore through better alerting and ensuring that technicians have the right tools and parts to resolve these issue as quickly as possible—hence, keeping downtime to a minimum.
The Internet of Things has created a new set of challenges for industrial organizations, but these organizations can use intelligent and effective data-analysis techniques to provide visibility for managers to drive the future of the IIoT going forward.
Nic Grange is the CTO of Retriever Communications. Nic has been with Retriever Communications since 2004 and currently serves as the company’s chief technology officer. His primary responsibilities include evaluating new technologies, particularly in the areas of mobile, automation, integration, security and the cloud.