Internet of Things (IoT) applications leverage the ever-growing volumes of data being generated by sensors, cars, and other connected or “smart” devices. Industrial IoT applications commonly embed sensors into a physical manufacturing process in order to detect potentially unsatisfactory conditions that could negatively impact the quality of goods being produced. Oil-drilling applications use data from sensors embedded on the drilling equipment in order to measure well output. Smart-city applications use data about vehicle positions in order to understand traffic patterns. In each of these examples, data streams, generated over time by many devices, are aggregated in a central data platform in order to gain insights to improve a complex system.
But not all IoT applications are created equal. Within the field broadly defined as the IoT, there are many distinct types of applications. One useful classification is to consider whether the data streams are collected and analyzed offline or are processed continuously in real time.
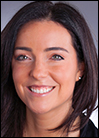
The former class of IoT applications, which I shall refer to as offline IoT applications, is not unlike traditional business intelligence or reporting applications. In an offline IoT application, data that is generated by sensors or devices is aggregated, stored and analyzed in a batch process in order to gain insights on the basis of potentially large volumes of data from disparate sources. The insights gained from offline applications are retrospective—they can describe what has happened in the past so that coarse-grain corrections can be made going forward.
The latter class of IoT applications, which I shall refer to as real-time IoT applications, processes data streams continuously in order to make operational adjustments in real time while a business process is taking place. By analyzing and acting on data as it is generated, a real-time IoT application can inform fine-grained optimizations that can yield dramatic efficiency gains for an ongoing process.
The value of real-time derives from the fact that the value of data decays over time. For a simple example of this principle, consider weather information. Knowing what the current weather conditions are has value because it can aid in planning what to wear or whether to pack an umbrella. Knowing what the weather was like a week ago is less useful in making decisions today. Similarly, knowing what current traffic conditions are like today is more valuable in planning a driving route than knowing what traffic conditions were like yesterday.
The same principle is true in IoT applications. For example, if sensors are measuring heat and vibration continuously during a manufacturing process, quality-control issues can be detected in real time and be resolved before they can impact the manufacturing operation greatly. By contrast, if sensor data from the manufacturing process is collected, stored and then analyzed offline only once a month, we can gain insights into how the manufacturing process is performing overall and can identify past inefficiencies, but we have no ability to intervene in order to improve the yield for the past month.
While real-time IoT applications create great opportunities, they present significant challenges from a technology perspective. A data platform capable of supporting a real-time IoT application not only requires the ability to ingest potentially large amounts of event-based device data, but must also support the fast analysis of that data and the ability to trigger an action should there be a need. Specifically, the ingestion of real-time sensor data requires a streaming data framework, the analysis of that data stream as it is generated requires a streaming analytical engine, and acting on that data might require an operational data store and a transactional engine.
Moreover, these various technologies must be fully integrated into a single unified platform in order to avoid the latencies associated with the movement of data between different systems. In short, in order for data to be ingested, analyzed and acted upon with low latency, a platform supporting real-time applications should converge multiple data and processing capabilities into a single, unified platform.
In the end, the true promise of the IoT is derived from the ability to create hyper-efficient business processes that can be continuously optimized. Although there are significantly more offline IoT applications than real-time applications at present, the field has passed an inflection point on the path to maturity. We now have exemplary examples of real-time IoT applications that demonstrate the potential of how these applications are driving value across industry verticals.
Computer chip manufacturers are increasing yield optimization in real time using sensor data from the manufacturing process, oil and gas companies are improving oil well output by adjusting the drilling process in real time, and smart cities can alleviate congestion by adjusting traffic light patterns. These applications go well beyond deriving offline insights in a batch process by automating the optimization of real systems without human intervention, and by leveraging data that is newly generated when it is most valuable.
Crystal Valentine is the VP of technology strategy at MapR Technologies, with a background in big-data research and practice. Before joining MapR, she was a professor of computer science at Amherst College. She is the author of various academic publications in the areas of algorithms, high-performance computing and computational biology, and holds a patent for extreme virtual memory. As a former consultant at Ab Initio Software working with Fortune 500 companies to design and implement high-throughput, mission-critical applications, and as a tech expert consulting for equity investors focused on technology, Dr. Valentine has developed significant business experience in the enterprise computing industry. She received her doctorate in computer science from Brown University and was a Fulbright Scholar to Italy.