The year 2018 was formative for Industrial Internet of Things (IIoT) technologies and deployments. Looking ahead to 2019, here are seven predictions for the commercial and industrial IoT, along with an outlook on computing size, the value of true edge computing, closed-loop edge-to-cloud machine learning and more.
1. Survival of the smallest: IIoT analytics and machine learning (ML) companies will be heavily measured on how much they can deliver in how little computing.
As IIoT projects extend beyond cloud-centric approaches, the next step in the evolution of artificial intelligence (AI) and the IIoT will address the need to convert algorithms to work at the edge in a dramatically smaller footprint. According to Gartner, 75 percent of enterprise-generated data will be processed at the edge (versus the cloud) within the next four years, up from less than 10 percent today. The move to the edge will be driven not only by the vast increase in data, but also the need for higher fidelity analysis, lower latency requirements, security issues and huge cost advantages.
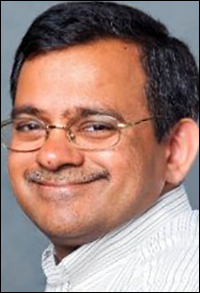
While the cloud is a good place to store data and train machine learning models, it cannot deliver high-fidelity real-time streaming data analysis. In contrast, edge technology can analyze all raw data and deliver the highest-fidelity analytics, and increase the likelihood of detecting anomalies, enabling immediate reaction. A test of success will be the amount of power or computing capability that can be achieved in the smallest footprint possible.
2. The market will understand “real” versus “fake” edge solutions.
As with all hot new technologies, the market has run away with the term “edge computing” without clear boundaries around what it constitutes in IIoT deployments. “Fake” edge solutions claim they can process data at the edge, but really rely on sending data back to the cloud for batch or micro-batch processing. When one reads about edge computing, the fakes are recognized as those without a complex event processor (CEP), which means latency is higher and the data remains “dirty,” making analytics much less accurate and ML models significantly compromised.
“Real” edge intelligence starts with a hyper-efficient CEP that cleanses, normalizes, filters, contextualizes and aligns “dirty” or raw streaming industrial data as it’s produced. In addition, a “real” edge solution includes integrated ML and AI capabilities, all embedded into the smallest (and largest) computing footprints. The CEP function should enable real-time, actionable analytics onsite at the industrial edge, with a user experience optimized for fast remediation by operational technology (OT) personnel. It also prepares the data for optimal ML/AI performance, generating the highest-quality predictive insights to drive asset performance and process improvements.
Real edge intelligence can yield enormous cost savings, as well as improved efficiencies and data insights for industrial organizations looking to embark on a true path toward digital transformation.
3. ML and AI models will become skinny with edgification.
Moving machine learning to the edge is not simply a matter of changing where the processing happens. The majority of ML models currently in use were designed with the assumption of cloud computing capacity, run time and computing. Since these assumptions do not hold true at the edge, ML models must be adapted for the new environment.
In other words, they need to be “edge-ified.” In 2019, “real edge” solutions will enable the relocating of the data pre- and post-processing from the ML models to a complex event processor, shrinking them by up to 80 percent and enabling the models to be pushed much closer to the data source. This process is called edgification, which will drive the adoption of more powerful edge computing and IIoT applications overall.
4. Closed-loop edge-to-cloud machine learning will become a true operational solution.
As ML and AI algorithms become edgified for use close to sensors or within IoT gateways or other industrial computing options, new best practices will emerge on how to train and further iterate on these models. What industrial organizations will find is that edge devices generating analytics on live streaming data (including audio and video) should regularly send insights back to the cloud, but only those that represent anomalous activity warranting a shift in the core algorithms.
These edge insights enhance the model, significantly improving its predictive capabilities. The tuned models are then pushed back to the end in a constant closed loop, reacting quickly to changing conditions and specifications, and generating much higher quality predictive insights to improve asset performance and process improvements.
5. Production IIoT applications will go into implementation only with edge-computing solutions supporting multi- and hybrid-cloud deployments.
Hybrid- and multi-cloud solutions will dominate industrial IIoT deployments; a recent report found that the hybrid-cloud market will reach US$97.64 billion by 2023. As industrial organizations look to bring multi-cloud environments together to provide a more cost-effective approaches and flexibility, it will be important for edge solutions to be cloud-agnostic.
Vendor-exclusive solutions will likely begin to fall by the wayside as companies look for more flexibility and freedom of choice when building their edge-to-cloud environments. Google, Amazon Web Services, Microsoft, C3 IoT, Uptake and other leading cloud providers will establish more collaborative partnerships with edge-computing companies to help businesses as they continue to improve and expand their offerings.
6. IoT video and audio sensors will take off, driving the need for deep learning at the edge.
There is industry-wide excitement about the capabilities that audio and video sensors can bring to the IIoT. Edge-computing technology can play an important role in the further deployment of audio and video data in commercial and industrial IoT systems. The fusing of asset data with audio and video analytics will allow for faster and more accurate device and machine maintenance (including updates on systems health and more), and a whole host of new innovative applications. One such example of video analytics is the use of flare monitoring at oil and gas operations to track environmental compliance and flare state remotely for large volumes of flare stack towers.
7. Predictive maintenance will give way to prescriptive maintenance.
One of the big promises IIoT edge solutions deliver is predictive maintenance, offering insight into what is likely to happen to a connected asset (like manufacturing equipment or an oil rig) in the future. While many organizations still lag in implementing predictive maintenance as a first step, even more advanced technology will be available to early adopters in 2019. Prescriptive maintenance is a step forward to enabling businesses to not only predict problems, but also produce outcome-focused recommendations for operations and maintenance using data analytics.
For example, elevator manufacturers want to put an end to routine problems, such as friction in elevator doors. As part of this effort, they can partner with FogHorn to create a predictive maintenance solution. By analyzing sensor data at the source, they can now determine maintenance needs well in advance, without the cost, latency, security and other issues associated with transfers of large amounts of data outside of a building.
Thus, a company can schedule service before anomalies impact performance in a highly efficient manner. As prescriptive maintenance becomes available, before the manufacturers roll a truck to provide maintenance on an elevator, they will have data available to suggest areas most likely to need repairs, and will have verified the repair staff person has the expertise, tools and parts available for the repair.
As FogHorn’s CTO, Sastry Malladi is responsible for and oversees all technology and product development. Sastry is a results-driven technology executive with deep technology and management experience of more than two and a half decades. Prior to his role at FogHorn, Sastry was the chief architect of StubHub, an eBay company, where he led the technology architecture transformation and also spearheaded big-data initiatives and data-driven decisions. Sastry was also a key technology executive at eBay, leading the technology re-platforming effort from its monolithic architecture to the distributed and scalable service-oriented architecture that it is today, enabling business growth. Prior to joining eBay, Sastry was co-founder and CTO of OpenGridSolutions, a founding member and architect at SpikeSource, and an architect at Oracle. He also worked at many other companies early in his career.