A team of researchers from the University of Michigan and the University of Washington have completed the first round of testing for an RFID-based system that uses UHF RFID signals to identify a specific tag, and that uses fluctuations in these signals to understand changes in the field around that tag. The result could be a solution with which users could understand whether a tagged item has been moved or been interacted with, as well as if someone has approached or left the tag’s vicinity.
The project, known as IDAct, has been underway throughout the 2018 to 2019 school year as researchers have worked to design a way to gain more information from a simple RFID tag read than just its ID number. The IDAct system takes advantage of received signal strength (RSSI), as well as RFID’s built in “phase” functionality, according to Alanson Sample, an associate professor at the University of Michigan and a co-author of the project’s white paper, titled “IDAct: Towards Unobtrusive Recognition of User Presence and Daily Activities.” The question Sample posed was how to turn basic UHF tags into little sensors. “The idea I had,” he says, “was to use a communication channel as a sensing mechanism.”
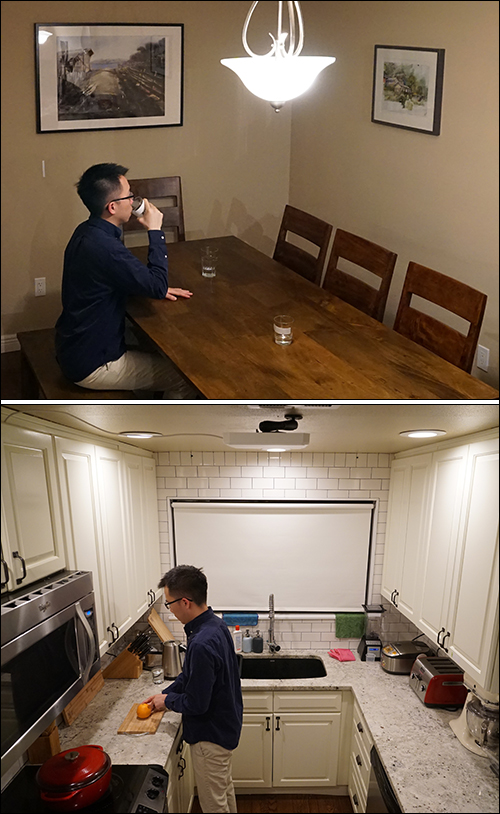
The system leverages changes in the RSSI and phase, as reported by the RFID reader, as well as machine learning, to infer what is happening around a tag. Traditionally, RFID readers and the software that manages the collected read data focus on receiving a unique ID number from a passive RFID tag, but they can also use RSSI to determine approximately where that tag is located, or the direction in which it is moving.
IDActs take that a step further by using the RF phase measurement. UHF RFID readers randomly change their transmission frequency across 50 channels, from 902 to 928 MHz, in order to meet FCC regulations and minimize interference with other devices. These changes in frequency take place at 0.2-second intervals. The phase difference between channels can be dramatically increased with motion, or due to a person’s presence.
The phase-sensing capability of RFID is what makes the IDAct system possible, Sample explains. One unique aspect of UHF RFID systems, he says, is that users can measure the phase of backscattered signals, which can be used to identify such details as a tag’s exact location; other technologies, such as Wi-Fi or Bluetooth Low Energy (BLE), lack a phase response.
The system has been tested in a dedicated apartment with 10 test volunteers, to detect such information as when a person entered or left a room and when he or she interacted with the tagged objects. The tags were attached to such items as toothbrushes, food packaging and pill bottles.
The team now plans to look for industry partners that could help to build out the technology for use in elder-care settings. Sample (from the University of Michigan) and Hanchuan Li (of the University of Washington) co-developed the technology with Shwetak Patel (also at the University of Washington) and Intel‘s Chieh-yih Wan and Raul Shal.
The IDAct project is initially aimed at elder-care environments to provide technology that would help caregivers, families or health-care providers view the health and well-being of an individual based on his or her daily activities. For instance, is that person brushing his or teeth, taking prescribed pills, exercising, preparing meals and so forth?
The technology offers other benefits beyond elder-care, the researchers note. Connecting everyday objects brings contextual awareness into everyday living, they explain, including tracking an individual’s hydration level by measuring the use of a water glass. And data from an object (a tagged yoga mat, for example) could prompt home-automation responses, such as playing exercise music or adjusting lighting each time a person stands on that mat.
For the test, the researchers installed Impinj Speedway R420 readers in several rooms of the volunteer’s apartment. The team then applied tags to 26 different household objects. For instance, they tagged the implements used to blend a smoothie, including a blender, a cutting board and a milk container. To track whether that individual made a sandwich, they tagged a cutting board, a plate and bread packaging, among other items. Altogether, the group classified 24 activities that the volunteer would undertake in the kitchen, dining room, living room and bathroom.
The solution then began making inferences. For instance, continuous motion of a mop in the kitchen led the system to infer that the individual was cleaning the floor. The researchers designed the technology to use near-field detection so that tags would experience interference of their transmission at a distance of 2 or 3 inches, while a reader’s firmware could detect subtle changes based on the presence of a person who was simply in the same room as the tag.
The group found, during testing, that the accuracy of activity detection was about 82.8 percent across all activities. To build a solution for commercial use, the researchers hope to partner with technology companies to develop software, as well as carry out the integration of the firmware and hardware required to enable the RFID reader to identify the changes in RSSI and phase. Sample envisions readers being installed in light fixtures in the homes or buildings of individuals whose activities are being tracked—something he has already tested with Intel and Disney Research.
Sample has been researching technological solutions for everyday life for years. His projects include ID-Sense, a human-object interaction detection system using RFID; RapID, a framework for fabricating low-latency interactive objects with RFID tags; and ID-Match, which is aimed at using RFID and computer vision to recognize individuals in groups (see Disney Research Explores Ways to Add RFID Intelligence to Robots, Toys).
According to Sample, future research will look into ways in which the number of RFID tags required to understand activities could be reduced. For instance, a tag attached to a wall within a specific room could allow the system to simply identify when an individual entered or exited that room, as well ashow active he or she was—for instance, whether that person was watching TV or exercising.
The IDAct pilot was completed this spring, and the researchers now plan to work with medical school partners to conduct further testing. Beyond health care and lifestyle management, Samples notes, the solution could be used in retail and other industries. For instance, if RFID tags were applied to goods at a store, readers could capture transmissions from the tags and identify how often a particular item on a specific display was picked up, along with how often that product was touched or when customers paused to look at it. That information could enable retailers or brands to better plan their displays to achieve greater sales rates.
In addition, the technology could be deployed in the gaming and toys industry. By applying an RFID tag to a toy and installing a reader, users could track information that could help a child with a toy interact with the digital world. For instance, a system capturing data from a tagged stuffed bear could detect when the doll was being hugged and present relevant content in a Web-based game, according to that action. The researchers also want to create open-source software with which developers could build their own systems to gain intelligence about what takes place within an RFID-enabled room.