The Internet of Things (IoT) is everywhere. The market is expected to grow from $170.57 billion in 2017 to $561.04 billion by 2022, at a compound annual growth rate (CAGR) of 26.9 percent, according to a June 2017 MarketsandMarkets research report. With growth comes challenges, especially in relation to technology.
According to the report, “The fundamental problem posed by the Internet of Things is that network power remains very centralized. Even in the era of the cloud, when you access data and services online you are most often communicating with relatively few massive datacenters that may or may not be located conveniently close to you.”
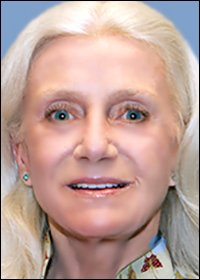
“That works when you are not accessing a lot of data and when latency is not a problem,” the report continues. “However, it does not work in the Internet of Things, where, for example, you are monitoring traffic at every intersection in a city to more intelligently route cars and avoid gridlock. In that instance, if you wait for the data to be sent to a data center hundreds of miles away, processed, and then send commands back to the streetlights, it is too late—the light has already changed.”
In addition, the Internet is a congested and often unreliable transport mechanism for time- and business-critical data delivery. Yet, according to IBM, we currently have 1 trillion devices exchanging 2.5 billion gigabytes per day over the Internet. However, computing location is only part of the story. Getting the right data from the right device at the right time is not just about hardware and sensor location—it is about data intelligence.
There are three core issues which must be addressed in order to solve the data-distribution dilemma. The first is latency. Information becomes irrelevant if the data does not get to the user fast enough. Time delays kill revenue opportunities in monetary transactions like e-gaming and stock trading, and cause loss if, for example, data from sensors on an oil rig are delayed, or patients can die if critical health information is not received. Seconds are no good. Data must be sent and received in milliseconds, often in volume and to thousands of recipients.
The second issue is efficiency (i.e. “one pipe in and one out”). The magic calculation is message size X message frequency X number of connections = throughput – how many gigabits of data per second can get through the pipe. If messages are smaller, you can either transmit more messages or hold more connections. Message size is the only factor in this equation that can be affected because applications must serve however many messages or connections are required in any given situation.
This brings us to the third issue, scale. You need to be able to scale over a busy and sometimes unreliable network. Many companies try to shoehorn inefficient enterprise messaging technology into their software, or use open-source and try to build their own. Unfortunately, organizations are trying to solve scalability issues using traditional techniques and solutions which weren’t built for the IoT world. These technologies don’t scale.
The IoT market is maturing, and with that comes a realization that network-efficient, high-volume data streaming and messaging is critical for corporate applications and analytics. Simply put, companies using IoT devices must have solutions that increase reliability, and reduce bandwidth and infrastructure requirements. This equates to intelligent data distribution and management, in conjunction with an architecture designed to put the data as close to the end user as possible—whether that is a machine, a device or a person.
In addition, to lighten the load on the network, companies need to understand their data. This means the technology they employ must apply intelligence and only distribute what is relevant or what has changed, so that only smaller pieces of data are sent across congested networks.
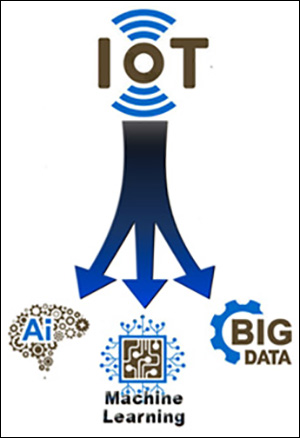
When approaching IoT data distribution, you cannot do so in the same way you conduct mobile data distribution. You need a strategy for collecting all the data from “things” at scale over unreliable networks, the intelligence to only pass on what is relevant, resilience to cope with sometimes unreliable networks and connections, and efficiency so you are not exhausting bandwidth. In some cases, data is sent to a warehouse for storage in the event of auditing or reporting, but some of the data must to go through your analytics engine, AI systems or real-time tools—for example, for fraud prevention or risk detection for processing.
Once processed, your data then needs to be distributed. You need to understand, at speed, what needs to be handled, then send it for processing and distribute it—whether the data is addressing the stoppage of a stolen credit card, telling first-responders to an accident that they need to change routes due to traffic congestion, or changing traffic light signals to reduce congestion.
The problem is that many data-communication technologies are messaging systems that blindly send large amounts of information back and forth. The specific demands of the IoT preclude effective use of general-purpose data-transmission technology solutions that work adequately for less demanding operational environments, such as chat or social-media platforms.
Therefore, intelligent data distribution is one answer. If you use technology that is both message-size-efficient and data-aware, you can intelligently, automatically and optimally manage data transmission and remove out-of-date and/or redundant data. This can deliver up to 90 percent data optimization across the Internet—which, in turn, translates to a substantial reduction in bandwidth and infrastructure requirements, and assures minimal latency for data transport.
If systems can understand data and only distribute what is important, at the application level, this is more powerful than any amount of hardware thrown at the problem.
Andréa Skov is an international marketing, sales, and operations executive with a successful 25-year career in strategic planning and innovative tactical execution to ramp high tech companies from inception through liquidity events. She is currently CMO of Push Technology Ltd. Her previous positions include chief marketing officer at Teneros (acquired by Ongoing Operations), CMO of All Covered (acquired by Konica/Minolta), president and founder of CoolSpeak (acquired by SunCorp Technologies) and CMO of ICVerify (acquired by CyberCash). Andréa holds BS and MS degrees in physics from Northeastern University.