It’s no secret that companies in every industry are rapidly embracing Internet of Things (IoT) solutions. But amidst all the hype, it’s easy to forget that IoT technologies have not yet reached maturity. In the rush to adoption, many manufacturers and other users have not fully considered security as part of their strategy. In IoT environments, security is not only about protecting data but also addressing vulnerabilities that are inherent in many IoT devices.
For example, with infusion pumps and other medical devices, the focus has often been on the primary functionality of the device, rather than on security. In a home or office, intelligent appliances often employ inexpensive, lightweight security protection that is not as robust as enterprise security. If a smart speaker or appliance is using a default username or password, or if it employs a weak authentication protocol, a hacker could hijack the device and use it as a gateway into a home or small business network. This type of risk is just the tip of the iceberg.
In “All Things Considered: An Analysis of IoT Devices on Home Networks” (Kumar, Deepak, et al. 28th USENIX Security Symposium, 2019), a large-scale empirical analysis revealed 83 million IoT devices in 16 million homes worldwide. A look at the security profiles of home appliances, game consoles, surveillance, wearables, voice assistants and other devices showed serious security concerns. Many devices used weak passwords and employed default administrative credentials that were often left untouched by users.
New Threats Require a New Security Approach
Despite growing security concerns, the good news is that regional and national governments worldwide are introducing legislation to help mitigate them. California’s new SB-327 law requires manufacturers to eliminate default passwords for devices, and the United Kingdom now has a certification scheme to label compliant devices with a “Secure By Design standard,” which could help manufacturers better brand security as a competitive differentiator.
Although government regulation is a strong first step toward improved security, there is still much more to be done as we adopt IoT security best practices. Artificial intelligence (AI) and machine learning (ML) can help by enabling us to predict threats. AI-enhanced approaches (Figure 1) will be able to go a step beyond spotting compromised devices and apply learning-based security techniques to proactively identify devices that could potentially present a risk.
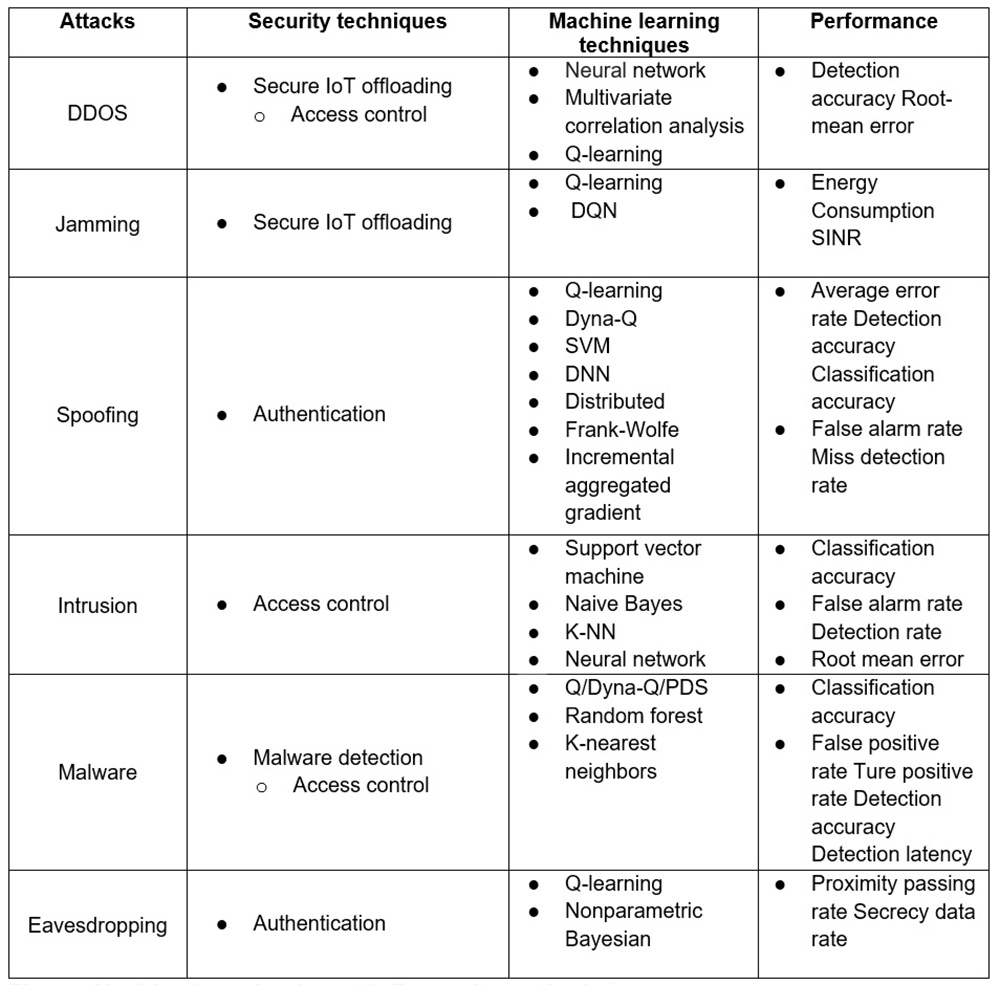
Figure 1: Machine learning-based IoT security methods. Source: Xiao, Liang, et al. “IoT Security Techniques Based on Machine Learning,” arXiv preprint arXiv:1801.06275 (2018).
Effective IoT security is based on identifying patterns by collecting data from a variety of devices over time. In larger IoT environments such as manufacturing, of the most significant challenges in IoT environments is the volume of data that is constantly being generated. This data is always changing, and it is challenging to manage with traditional analytics tools.
Techniques driven from AI and ML can provide support for different analytic methods toward spotting variations and abnormalities in large-scale data. For example, a properly trained ML algorithm could identify a suspicious data-traffic pattern and proactively alert an IT or security team, without the need for human intervention. Granted, there will be a training phase during which an IT individual would require to label malicious vs. normal traffic.
AI could also be used to enhance physical security for business campuses and other environments. For example, by collecting data from environmental sensors in a building, along with facial recognition data from connected cameras, an organization could gain insights into whether an authorized person has gained entry. That being said, privacy plays a big factor in this approach. Working together, surveillance systems and AI applications could compare real-time images to a database to control access to sensitive areas.
Staying a Step Ahead of Cybercriminals
Ultimately, understanding and stopping IoT attacks requires collecting massive volumes of data, analyzing it closely to determine what activity is malicious, and employing effective planning, proper deployment and thorough monitoring. New technologies and vulnerabilities are constantly under development, and AI and ML can assist in identifying vulnerabilities before they are exploited.
Avesta Hojjati is the head of R&D at DigiCert, where he manages advanced development of cybersecurity products. Before joining DigiCert, Hojjati was part of the Symantec and Yahoo security teams, as well as operating his own cybersecurity startup. He focuses on applied cryptography, blockchain, post-quantum crypto and IoT security. Hojjati earned his master’s degree in computer science with a concentration on security from the University of Illinois at Urbana Champaign, and he recently completed his PhD dissertation on applications of blockchain and IoT in manufacturing.