More than 8 billion connected devices were reportedly in use last year, up 31 percent from 2016, according to a Gartner research report. The same report states this number will increase to 20.4 billion by 2020, with devices ranging from cars to cameras.
The numbers point to a fundamental shift in the way consumers engage and interact with each other—including the desire for more innovative ways to pay for goods and services. Businesses trying to improve their customer engagement must meet their customers “where they are,” by offering preferred payment methods and ensuring these methods are both as simple and as secure as possible—historically contradictory requirements.
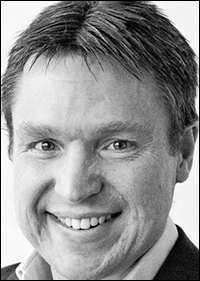
In an environment in which people can pay with the tap of a phone or from a wearable device, there’s also an inherent consumer expectation of faster and more secure payments. Unfortunately, the fast-paced payments process is opening channels for faster fraud, with the Internet of Things (IoT) providing fraudsters with an abundance of new devices to use as channels for criminal activity.
For some industries, the growing creation and adoption of IoT devices equates to the need to further develop and execute strategies to detect and deter fraud. Enter adaptive behavioral analytics and real-time machine learning.
When looking at how behavioral analytics can assist with risk-mitigation strategies as a consumer, we can look to the example of voice-assistance technology. In a true fraud situation, if you are out of town and leave a window cracked open and your voice-activated device visible, a thief can easily order items, have them shipped to your home and pick them up before you even return. With advanced behavioral analytics, your method of payment would realize you are traveling and flag the order as fraudulent.
There are also situations which are not as nefarious, but can easily occur in the same scenario. If you have background noise while ordering windshield wipers, your technology could hear “Order baby diapers.” Machine learning can pick up on your patterns and realize you don’t order diapers, and alert you that this could have been an order placed in error.
While machine learning and behavioral analytics have been around for years, many industry experts are predicting 2018 will be its momentous point. According to Gartner’s latest CIO survey, respondents indicated machine learning and artificial intelligence are in their top-five priorities this year, with 21 percent of CIOs from around the world testing or considering these initiatives in the short term. One quarter of the CIO respondents have medium- or long-term plans for the technology. There are many reasons behavioral analytics should be an integral part of the short- and long-term technology roadmaps, especially for financial institutions and payments processors.
The Reality of Everything
One primary reason is today’s fraud reality. We don’t need to look any further than our daily news briefs to see the impacts of data security breaches. Fraudsters will continue to seek the greatest opportunities for data compromise and locate the weakest link in your risk-mitigation strategy.
The more the industry adapts to changing fraud patterns, the more fraudsters adapt their individual activities to avoid detection. Retailers, banks and other businesses are actively seeking ways to combat this reality while avoiding poor payment experiences for their customers—including failing to convert a customer because of cumbersome false declines on their genuine transactions.
Businesses can’t seem to crack the problem. This is where adaptive behavioral analytics can play a key role. By detecting irregularities in real time, it allows users (especially banks and payments processors) to identify new types of transactional fraud the moment it occurs. Over time, this information reduces false positive alerts and enables banks to accept their customers’ genuine transactions. These financial institutions are better equipped to understand anomalies because they truly understand their customers’ legitimate individual behavior.
Machine learning also has the adaptive ability to self-learn, thereby reducing the need for manual intervention—a luxury we don’t have in the need for faster payment. Because it individualizes the customer experience, you can reduce friction in your customers’ payment experiences while recognizing operational efficiencies with your internal processes. Real-time analytics can simultaneously meet two important needs for the payments arena—improved customer service and operational cost reduction.
As the IoT allows the world to become more connected, the outlets and devices we trust must be coupled with the best technology to deliver the most secure environment possible. Machine learning will continue to play a critical role in identifying how users in this ecosystem operate and where anomalies exist.
Faster and easier payments can often come at a risk. Machine learning can simplify our understanding of those behaviors that lie outside the user’s normal patterns—allowing the IoT to know and understand you as an individual.
David Excell is Featurespace‘s founder and CTO. Dave is an experienced technology innovator with more than 18 years dedicated to the study of engineering. He has received several awards in his field, including the ITC Enterprise Award for Young Entrepreneur. Under his leadership, Featurespace has progressed from a concept to a commercial success. Dave currently resides in the United States and is managing the Featurespace global expansion from the company’s U.S. headquarters in Atlanta, Ga.